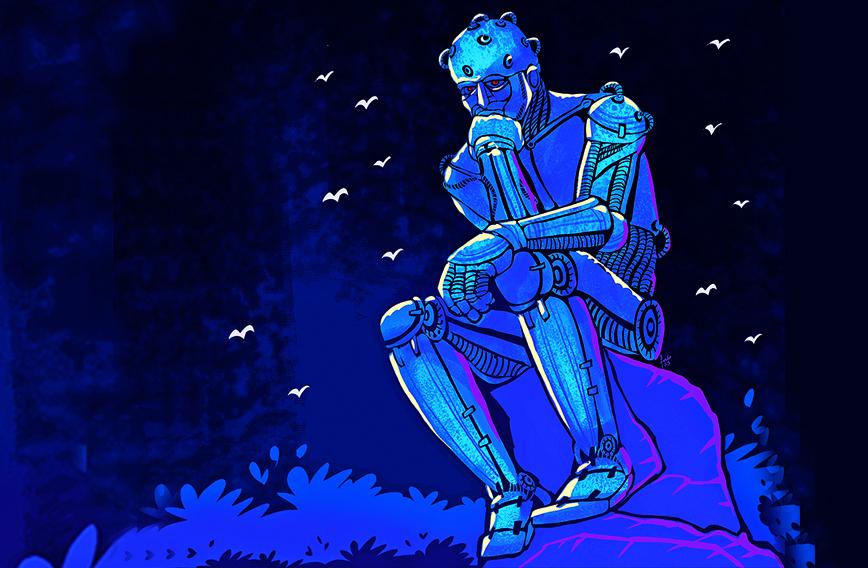
Being in the age of AI
By Kiran Karnik
JULY 16, 1945, marked the start of a new era in human history: the beginning of the nuclear age. On that date, in Alamogordo, New Mexico, a nuclear device was successfully tested by the US. Watching the test, Robert Oppenheimer (then director of the Los Alamos laboratory, where the bomb was created) — presumably in awe, and possibly in fear — quoted the Bhagavad Gita: “Now I am become death, the destroyer of worlds.” Less than a month later, the first nuclear bomb was dropped on Hiroshima, causing unimagined devastation: truly, the destroyer of worlds.
This was followed, a few days later, by another nuclear bomb dropped on Nagasaki; these twin bombings have so far been their only use in destruction. However, ever more sophisticated and powerful nuclear devices (including hydrogen or fusion bombs) have been developed, tested, and deployed, with the worldwide inventory of these estimated as exceeding 12,000. Even a fraction of these can wipe out much of the life on earth. In 1945, the genie was out of the bottle and, like toothpaste out of a tube, can never be put back.
Are we now in the process of letting another genie out of the bottle, in the form of Artificial Intelligence? The first nuclear test was preceded by many years of theoretical physics (Einstein’s simple equation, E=mc2 — the basis of nuclear energy and bombs — was formulated in 1905), developments in other related fields, and practical experiments on splitting the atom. Similarly, today’s AI has its genesis in many years of foundational work in various disciplines, converging into user apps like ChatGPT. Are we then on the threshold of another new era in human history — the AI Age — or have we already crossed the Rubicon?
To many, the analogy with the nuclear age would appear strange. After all, ever-more-capable computers, and increasingly sophisticated software and applications, have been positive developments. They have made life easier, speeded up processes, and brought in transparency. In conjunction with communication technology, they have revolutionized work, entertainment, connectivity. Information of all kinds is now available anywhere, anytime, and literally at your fingertip, on a computer or mobile phone. All you have to do is to articulate a question (no literacy required) into your mobile phone — in one of many languages — and you will get a near-instantaneous response.
Despite all its wondrous applications, AI has a potentially dark side too. It is this that is of growing concern, worldwide. Evidence of these worries is seen even in the public sphere. Some weeks ago, a number of tech leaders, including Elon Musk, sought a six-month “pause” in the development of AI, so as to allow time for formulation of a regulatory framework. Physicist and Nobel Laureate Stephen Hawking too had apparently expressed deep concern about AI and its implications. The CEO of OpenAI, developer of ChatGPT, has himself called for regulations on AI. US Vice-President Kamala Harris convened a meeting of CEOs of big tech companies to discuss AI and its impact.
Though the US seems to favour minimal state intervention for the moment, the European Union is already drafting a law to regulate AI; meanwhile, Italy has gone a step further and banned ChatGPT, mainly due to worries about its access to private data. China already has tight control on the internet and digital technologies; doubtless, it will soon have laws in place to deal with the use of AI.
In India, discussions about possible regulation are underway. The Digital Data Protection Act, now being drafted, will certainly cover some aspects. A minister has stated that the proposed Digital India Act (a revamp of the Information Technology Act) will have a separate section on AI and other emerging technologies, and will focus on protecting users. Aspects like fake news are to be handled through a government-created mechanism that can order the taking down of any item.
GROWING AT WARP SPEED
So, what is the “dark side” that has rather suddenly spawned so much worry, worldwide, about AI? More on these worries later, but the trigger has clearly been the advent of ChatGPT, which has propelled AI from the background — embedded mainly in business-to-business software — into a consumer product that is available for every smartphone. Its popularity, indicative of its tremendous functionality, can be gauged from the fact that the app had over a million users in just five days (Facebook took 10 months and Twitter two years), and over 100 million in two months, making it the fastest-growing app in history. Even the sensational Tik Tok took nine months to reach the latter milestone.
The autonomous car is here to stay
As much as the warp-speed growth of the app, what has thinkers worried is the capability of its foundational software, GPT or Generative Pre-trained Transformer and, more broadly, generative AI. So, what exactly is it and why have so many expressed concerns about it? The journey began about a decade ago, with “deep learning”, which used vast data bases and neural networks running on powerful computers, to recognize images, process audio and play games: the latter with human-superior capability. For example, Alphabet’s AlphaGo software beat a top player of the Chinese game Go in 2016. In fact, the potential of machine learning was exhibited as far back as 1997, when IBM’s Deep Blue defeated chess grandmaster Garry Kasparov.
Building on deep learning, now so-called large language models (LLMs) draw on massive datasets and underpin an app like ChatGPT. Its working can be simplistically explained by taking the example of asking it to complete an unfinished sentence. The LLM first converts each word or word-group into a pre-assigned number (or “token”); each is then put in a space along with other similar words. Next, based on its training, its “attention network” links related words (e.g., which noun does the adjective qualify: “beautiful” being linked with “roses” in “a beautiful collection of roses”); it then picks the highest probability word to find the next word in an incomplete sentence; finally, it goes through iterations (autoregression), based on its training, to keep checking upto its limit.
The critical element is the size of its training database. One of the points of concern regarding AI is that as these LLMs grow larger, their capability increases even faster — but unknown quirks sometimes come up. One measure of growth is the difference between GPT-3 and the next version, GPT-4, developed just a few months later. The former could process up to 2,048 tokens at a time; GPT-4 can handle 32,000. As an instance of how this increases capability, take the American Uniform Bar Examination: while GPT-3.5 (superior to GPT-3) failed, GPT-4 passed it by being in the 90th percentile. The combination of faster computers, massive databases and better algorithms that draw and learn from them are the drivers for LLMs.
GPT-3 drew from data available on the entire internet from 2016 to 2019, selecting a very small subset of it, after filtering out the loads of junk, for its training. GPT-4 was trained, according to reports, on a vast base of images too. The large databases are necessary for self-learning by LLMs. This follows the simple methodology of a self-test: taking part of a text and trying to guess what words would complete it; checking its answer against the original text enables it to learn on its own. Obviously, the more such “tests” it takes and learns from, and the bigger the size of the data it can draw on, the more accurate it becomes. Hence the need for super-fast computers and really large databases. In practice, the LLM does this by operating various “attention networks” in parallel, enabling scaling, but requiring computers with sophisticated Graphic Processing Units (GPUs).
While the growth in capability from one generation of AI to the next (GPT-3 to GPT-4) is exponential, so is the requirement for computing power (as also electric power and skilled human-power) — and hence cost. One estimate indicates that training GPT-3 cost $4.6 million; this escalated to the order of $100 million for GPT-4. Governments and companies may not be deterred by costs of this order, so the constraining factor is more likely to be the amount of data available: we may soon reach the limit of high-quality text which can be downloaded from the internet. Also, without some major breakthrough on computer hardware, speed of data processing may become a constraint too.
COMPANIES RUSH IN
Meanwhile, companies are rushing to use the new technology in all their applications: not only for near-instantaneously digging out any information which may be required, but also conveying it through chatbots that can now emulate human response (leave alone speech) so well that the human-machine differentiation has become very difficult. Many routine tasks are being automated, making for greater efficiency, speed, lower costs, and reliability — as also 24x7 availability. Apart from completing sentences and scouring databases to answer queries, AI can now write reports, essays and poetry, or create illustrations and sketches, amongst other things. Generative AI (GAI) is very definitely the top-of-mind, flavour-of-the-year topic in boardrooms around the world.
GAI’s capabilities are not only spreading rapidly and extensively in the sphere of business and industry, but also to health, education, scientific research and a wide range of other areas. In education, it has opened up vast possibilities: once more, there are dreams of creating a system that is equitable, extensive, and excellent. Yet, even as the ways AI can best be used in learning are being explored, the flip side of it being used to “cheat” (e.g., ChatGPT being used to write essays and assignments) are already common. Now, much energy and skill are going into devising ways of using AI itself to spot such unethical practices. As in many other fields, technology is both the problem and the solution, with the two bound in a neverending cycle.
At the same time, AI in combination with electronics and robotics is changing the shop floor in factories, the operation theatre in hospitals, and the laboratories and classrooms in educational institutions. In conjunction with sensors and machine-to-machine communication, it can ensure safety and efficiency through predictive analytics that will ensure on-time maintenance of machines, equipment, vehicles, planes and ships. The marriage of hardware and software is becoming more ubiquitous, extending to a variety of problems in different fields.
A few days ago, there was news of a person who, as a result of an accident in 2011, was paralyzed below the waist, and told he would never walk again. However, as reported in Nature, a digital interface developed by scientists in Switzerland has now restored communication between his brain and spinal cord, allowing him to stand, walk and even climb stairs. Chip implants in humans will soon become commonplace; software and AI may enable them to replace, supplement and augment various bodily functions and organs, enabling human capabilities to reach new levels. With Musk’s Neuralink receiving a green light from the US Food and Drug Administration to go ahead with in-human clinical trials for a brain implant, one can imagine this going to the next level as a brain to machine link is established and AI brought into play.
Hundreds of other uses are already underway and many hundreds more will certainly be seen as innovators begin to leverage the endless possibilities of GAI. Indicative of the tremendous surge in the actual use of AI is the speed with which ChatGPT has spread. Another is the present and projected growth in demand for the necessary hardware. This is manifested in the market cap of Nvidia, by far the biggest maker of the chips and GPUs which power the fast computers necessary for LLMs and AI. In just one day, on May 25, its market cap rocketed by a mind-boggling $184 billion, taking it to just short of a trillion dollars (a landmark it has now crossed). Open-source implementation of LLMs is now on the cards and many innovative new apps will certainly ride on these, further boosting demand.
CHEAPER THAN HUMAN LABOUR
The increasing capabilities of GAI mean that it can now perform many functions being done by humans. Its ability to do this at a cost lower than human labour, and to do so efficiently and autonomously, is likely to result in a new transition: the “outsourcing” of work to machines. In the short term, jobs are likely to be lost, though historical evidence points to the fact that every technological breakthrough has resulted in ultimately creating more (though different) jobs. However, apart from increased unemployment in the short term, there will be pain for many who do not have — and may not be able to pick up — the skills required for the new jobs that will result. Also, there is deep concern that AI is different and that job losses will be both permanent and massive. Of course, every new technology claims such exceptionalism. Is AI truly going to be different? Some give it a “co-pilot” role, implying continuing need for human involvement. However, this will not obviate the need for fewer humans, as AI drives the efficiency of output.
![]() |
A doctored picture of the wrestlers smiling |
Many are concerned about the even broader issues that may result from the human-comparable (possibly super-human) capabilities of AI. It is this that is the basis of worries raised by experts. Science fiction stories sometimes are built around a future in which machines and robots take over the world. As AI becomes ever more versatile and powerful, will it become more autonomous? Already one has practical scenarios where it makes sense for a machine to override humans. One example is a car facing a sudden obstacle: an AI-driven computer can more quickly sense and process all the data to decide on the optimum action — brake, swerve, etc. — and also act faster than a human.
As instances like this multiply, will most decision-making pass on to machines? Will humans become a species inferior to machines? Will AI do to humans what we have done to other species — including driving many to near-extinction? Should we be paying serious attention to a recent letter, signed by over 350 experts, including Sam Altman, CEO of OpenAI (the creator of ChatGPT), which warns of “the risk of extinction from AI”, suggesting that it should be “a global priority alongside other societal-scale risks such as pandemics and nuclear war”?
The many broader aspects of AI and its impact have taken discussions of this from the corporate boards to Cabinet meetings in many countries. The focus is not only on how it may be used — by companies, institutions, and countries — but also on concerns about its impact: on jobs, on security, and through its use by much of the population (via new apps like ChatGPT, for example, or by its embedding in existing apps).
These and the larger questions noted earlier are no longer merely speculative or philosophical, nor are they of some distant future. We need to ponder them with some urgency, forgetting the competitive one-upmanship between nations. Meanwhile, even more immediate issues confront us, resulting from the power and versatility of GAI.
BIASES CREEP IN
One concern about AI, particularly for countries like India, is the bias that unintentionally creeps into the apps of which it is the foundation. Whether it is LLMs trained on text, or algorithms which learn from images, a very large proportion of the data used originates from the West, as do the algorithms themselves. As a result, the skewed data and biases of the programmer produce output that is discriminatory, or even wrong, in some situations. One simple example is with regard to the problem facing an autonomous vehicle when confronted with a choice of whether to avoid a child who suddenly comes in the way but then having to hit an older person. The decision algorithm fed into the computer depends on the view or bias of the programmer. In the case of training databases, an example is of face recognition: due to the images used (mostly “white” Westerners), its veracity depends on skin colour, being high for whites but sometimes making mistakes for dark-skinned people. One researcher documented this recently, explaining how, in 1998 — as a doctoral student — he had created an algorithm that unintentionally had a strong racial (colour) bias. The same bias now occurs in gender identification, where dark-skinned women are often identified as male. There may be such biases built into many apps because of the algorithm and the training data bases (using more balanced data is constrained by availability, which also results in greater cost). They are embedded deep within the AI system, and so are not easy to find.
Data-driven biases or those due to programmers’ perspectives are not limited to the West. LLMs which use Indian languages are prone to biases too, for the same reason of skewed data; similarly for apps developed here. In fact, given the historical problems in Indian society, texts used for training are more likely to be gender-biased, besides having possible caste biases. With the extensive inequity in India, images used for training are not likely to be representative of the vast diversity in the country. Mistakes and wrong identification are, therefore, likely. With the huge increase in surveillance cameras, and their use for face identification (not only of known criminals, but also of “trouble-makers”), the potential for harm is substantial. Inevitably, hackers may try to intentionally embed biases against specific individuals or segments of the population. These worries make it worth considering whether every app should mandatorily be required to go through some form of a “bias test”.
SMILING WRESTLERS?
Biases apart, while the capabilities of GAI are most impressive, it seems that LLMs are unpredictable and sometimes generate wrong and false information. With increasing size, capabilities — but also complexities and unexpected responses — increase rapidly. Their creative abilities can make things worse. One example is a recent incident in a Manhattan court. The lawyers of a litigant filed a brief which quoted more than half a dozen relevant court decisions which supported their contention, complete in most cases with details like the court and judges, docket number and dates. However, neither the opposing lawyers nor the judge himself could find the decisions and quotations summarized by the lawyer who had prepared the brief. In response, he admitted that he had used ChatGPT to do his legal research. Apparently, it had basically invented the judgments and details!
Closer home, some days ago there were painful images of forceful eviction, dragging, and detention by the police of wrestlers protesting at Jantar Mantar in Delhi. As these were circulating on social media, accompanied by critical comments about police high-handedness, a very contrary one popped up showing two top women wrestlers — key protesters — smiling happily while taking a selfie with the police. This strange image turned out to be fake, generated with great realism by AI. Similarly, probably as a joke, someone created and posted a false image of the Pope in a fancy puffer jacket. Many such fake images, rendered very realistic by AI, are now beginning to appear, supplementing fake news posts — and often giving apparent credibility to them.
Social media is already a major source for disinformation and fake news. Organized groups and bots are known to spread negative messages on a mass scale. The situation is made worse by the algorithms in play that feed news and views to selected individuals, reinforcing existing opinions and creating an echo chamber effect. The capabilities of AI to locate like-minded individuals and feed their biases will make this more serious, especially with the addition of images which are “realistic fakes”. On the other hand, AI may be trained and used to filter out any dissenting views or to specifically identify individuals who tend to be deviant — possibly (inevitably?) pre-emptively.
Another concern is the further heft that GAI will add to the growing power of big tech. Will a few companies dominate and — maybe literally — rule the world? Alternatively, or through these companies, will a few countries call the shots? Many are surprised that tech companies are themselves calling for regulation. One could ascribe this to an unexpected rise in their social consciousness, or a clever and selfish motive to maintain their leadership in this area through a freeze in development (by other companies/countries).
LOOKING AHEAD IN INDIA
As noted, individual countries, including India, are debating regulatory frameworks. The trade-off between regulation that is too early (throttling innovation) and too late (the horse may already have bolted from the stable) is a difficult one. India may be well served by resisting the temptation of showing it is a leader by being quickly off the blocks. A more measured approach is desirable, with a step-by-step roll-out that takes account of feedback from each move forward. Little or light regulation of research, more for platforms, and fairly stringent laws for apps may be a wise approach. Guidelines and guard rails might be a good start, before getting to mandatory regulations and laws.
Meanwhile, we cannot afford to be left behind in this key technology that already has visible ramifications in all areas: from daily life to economic efficiency, social sector uses, and security. India’s capabilities in hardware development, especially at the basic stages (beginning with chips/GPUs) is rather rudimentary. Our expertise — as in many other sectors — lies in the software, apps, and business models. This is an area in which we should push ahead with rapidity, and one in which we could have ambitions of being in the top bracket. It will require large investments, especially in hardware, data centres, and development of human resources.
Importantly, to maximize benefits and ensure that development is inclusive, there is need to create multi-lingual access, at least in major Indian languages. This requires large datasets for training, so as to ensure better accuracy and also quicker response. In this respect, non-English languages face a serious problem, because of the lack of digitally stored data that the LLM can feed on. This means greater processing cost, because it requires more “tokens” (one experiment showed that Malayalam required 15 times that required for English; another showed Hindi required almost five times). One laudable effort being made in India is by AI4Bharat, an IIT Madras initiative, which aims at “building an open-source language AI for Indian languages, including datasets, models, and applications”. Expanding such work will help to make GAI and its apps more inclusive. However, in doing this, we need to be cognizant of the dangers of bias that could creep in.
Despite the many worries, GAI holds great promise as a technology that might well compare with the steam engine or with the computer, in terms of impact. Policies need to be put in place which ensure that it does not widen inequity (within and between nations), or leave behind those who are disadvantaged, as other technologies have often done. A global regulatory regime may well be required, but it should be one that is not discriminatory and treats this technology as a global public good. As in a pandemic, none is safe until all are.
Are the concerns about AI’s negative impacts exaggerated? Will even the good it does result in an idle human race, with all work and tasks automated? Everyone loves a vacation, but will a permanent, life-long “vacation” be fun? Elsewhere (see 'Zero Sum Game,' Civil Society, May 2023), we have argued that some human functions will not be taken over by AI, that human “stupidity” will be a match for artificial intelligence. The future could then be human plus machine, rather than one or the other. Such a combination could end up being not a mixture, but a composite, taking the form of implants in humans, linked to a computer running GAI. This could result in GAI expanded differently: not generative AI, but Gen AI, like Gen Z (after all, in a circular world, A follows Z), a new generation, with brains and physique amplified by chips and AI. The question of who is in charge — human or machine — will not arise; having made some birds and animals extinct, humans may now have created an altogether new life form: Gen AI may not just be a new generation, but an altogether new species.
Kiran Karnik is a public policy analyst and author. His most recent book is ‘Decisive Decade: India 2030, Gazelle or Hippo’.
Comments
Currently there are no Comments. Be first to write a comment!